The Essential Role of **Machine Learning Labeling Tools** in Data Annotation
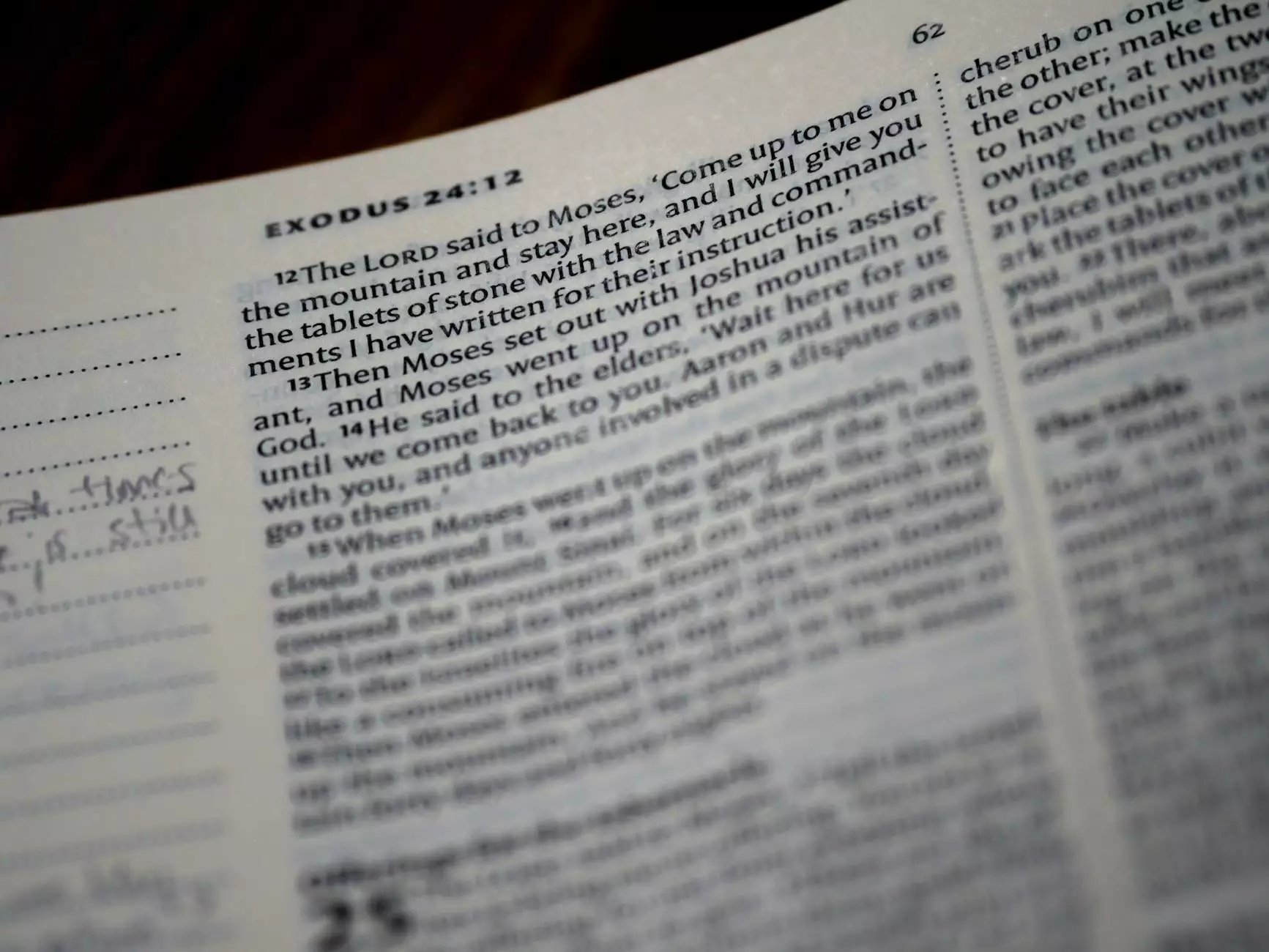
In the world of artificial intelligence (AI) and machine learning, data is the cornerstone of success. The accurate and effective labeling of data is crucial for training reliable AI models. This is where machine learning labeling tools come into play, revolutionizing the way data is annotated and prepared for use in various applications.
Understanding Data Annotation and Its Importance
Data annotation is the process of labeling data so that it can be easily understood and used by machine learning algorithms. The quality of data annotation has a direct impact on the performance of machine learning models. Without high-quality labeled data, AI systems may struggle with accuracy and reliability.
Types of Data Annotation
- Image Annotation: Used primarily in computer vision tasks, this involves labeling objects within images.
- Text Annotation: Commonly utilized in natural language processing, this includes tasks such as sentiment analysis and entity recognition.
- Audio Annotation: Involves transcribing audio data and labeling the elements for speech recognition applications.
- Video Annotation: Labeling frames in a video to help identify actions or objects over time.
A high-quality data annotation tool or platform can significantly enhance the speed and accuracy of these processes, allowing businesses to achieve their AI objectives more effectively.
How Machine Learning Labeling Tools Work
Machine learning labeling tools leverage advanced algorithms and user-friendly interfaces to facilitate data annotation. Here is how they function:
1. Data Uploading
Users can upload large datasets (images, text, audio, etc.) into the platform. Advanced systems often support bulk uploading for efficiency.
2. Annotation Techniques
These tools offer various methods for annotation, including:
- Manual Annotation: Where human expertise plays a crucial role in accurately labeling data.
- Automated Annotation: Utilizing pre-trained AI models to suggest labels, thus speeding up the process.
- Collaborative Annotation: Allowing multiple users to work simultaneously on annotating datasets, enhancing productivity.
3. Quality Assurance
Most platforms implement quality checks, where annotated data is reviewed for accuracy. This can involve:
- Automated Checks: Systems that flag inconsistencies or errors during the annotation process.
- Human Review: Assigning expert reviewers to assess and refine annotations for higher fidelity.
Features of an Effective Machine Learning Labeling Tool
When selecting a machine learning labeling tool, it’s crucial to consider the following features that contribute to efficient data annotation:
1. User-Friendly Interface
The best tools provide intuitive designs that allow users to navigate easily, requiring minimal training for new users. This includes drag-and-drop features, interactive tutorials, and customizable settings.
2. Support for Multiple Data Formats
Versatility is key. A good labeling tool should support a wide range of data formats, including images (JPEG, PNG), text files (TXT, CSV), audio files (WAV, MP3), and video formats (MP4, AVI).
3. Scalability
Your chosen tool should be scalable to accommodate growing data needs. Whether you have hundreds or millions of data points, the tool should handle increasing volumes without compromising performance.
4. Integrations
The ability to integrate with other software solutions, such as cloud storage services, project management tools, and machine learning platforms, is a significant advantage. This facilitates a seamless workflow from data collection to model training.
Benefits of Using Machine Learning Labeling Tools
Implementing effective machine learning labeling tools can offer a multitude of benefits:
1. Increased Efficiency
Automated processes drastically cut down the time required for data annotation, enabling teams to focus on more critical tasks while the tool handles repetitive labeling work.
2. Enhanced Accuracy
With robust quality assurance mechanisms in place, businesses can ensure their labeled data is of the highest quality, thus enhancing the accuracy of their models and reducing errors in deployment.
3. Improved Collaboration
Many machine learning labeling platforms allow multiple team members to work on projects simultaneously, facilitating collaboration and expertise sharing, which is essential in a fast-paced AI environment.
4. Cost-Effectiveness
By reducing the time and resources spent on data annotation, organizations can allocate budgets more effectively, potentially leading to significant cost savings in the long run.
Best Practices for Effective Data Annotation
1. Define Clear Guidelines
Establish precise guidelines for annotation tasks to ensure consistency across your dataset. Clear instructions help annotators understand expectations and outcomes.
2. Use Pre-Training Data
Utilize pre-trained models where applicable to assist with automatic labeling tasks, thus providing a baseline for manual editing and refinement.
3. Regular Training for Annotators
Regular training sessions for annotators can significantly improve the quality of their work. Providing knowledge on the latest techniques and tools establishes a culture of quality.
4. Monitor and Update Processes
Continuously monitor annotation processes and outcomes. Collect feedback to make necessary adjustments, allowing for ongoing improvement of quality and efficiency.
Real-World Applications of Machine Learning Labeling Tools
Machine learning labeling tools find application in various industries. Here are a few notable examples:
1. Healthcare
In the medical field, accurate data labeling can help train algorithms for diagnostic imaging, such as detecting tumors in X-rays or CT scans. This enhances clinicians' diagnostic capabilities and improves patient outcomes.
2. Autonomous Vehicles
Self-driving cars rely heavily on labeled datasets to interpret their surroundings. Machine learning labeling tools are crucial in annotating images and videos that feed into vehicle AI systems, improving safety and reliability.
3. Retail and E-commerce
In retail, data annotation assists in product recognition and inventory management. By labeling images and text data, businesses can leverage AI for better customer service and personalized shopping experiences.
4. Social Media and Content Moderation
Social media platforms use machine learning labeling tools to evaluate and categorize user-generated content. Accurate labeling helps in identifying inappropriate content and improving user experience.
Choosing the Right Machine Learning Labeling Tool
Selecting the right machine learning labeling tool can be a game-changer for your data annotation needs. Here’s a guide to evaluating options:
1. Assess Your Needs
Identify the types of data you will be annotating and your specific requirements. Consider factors such as volume of data, types of annotation required, and your team's expertise.
2. Read Reviews and Case Studies
Look for reviews and case studies from companies similar to yours. This can provide insights into the tool’s performance and reliability in real-world applications.
3. Request Demos
Most reputable vendors offer demos or trials of their products. Utilize these opportunities to test the tool’s functionality and ease of use before making a commitment.
4. Evaluate Support and Training
Choose a vendor that provides strong customer support and comprehensive training resources. This can be particularly beneficial for onboarding new users.
Conclusion: Unlocking the Future with Machine Learning Labeling Tools
In conclusion, machine learning labeling tools are pivotal in the evolving landscape of AI and machine learning. By facilitating high-quality data annotation, these tools empower businesses to create effective machine learning models that can transform industries. As technology advances, staying ahead in utilizing these tools will be essential for any organization aiming to leverage data for competitive advantage.
At KeyLabs.ai, we are committed to providing cutting-edge solutions in the realm of data annotation and labeling tools. Harness the power of machine learning and elevate your data processes today!