The Revolutionary Role of Malware Machine Learning in IT Security
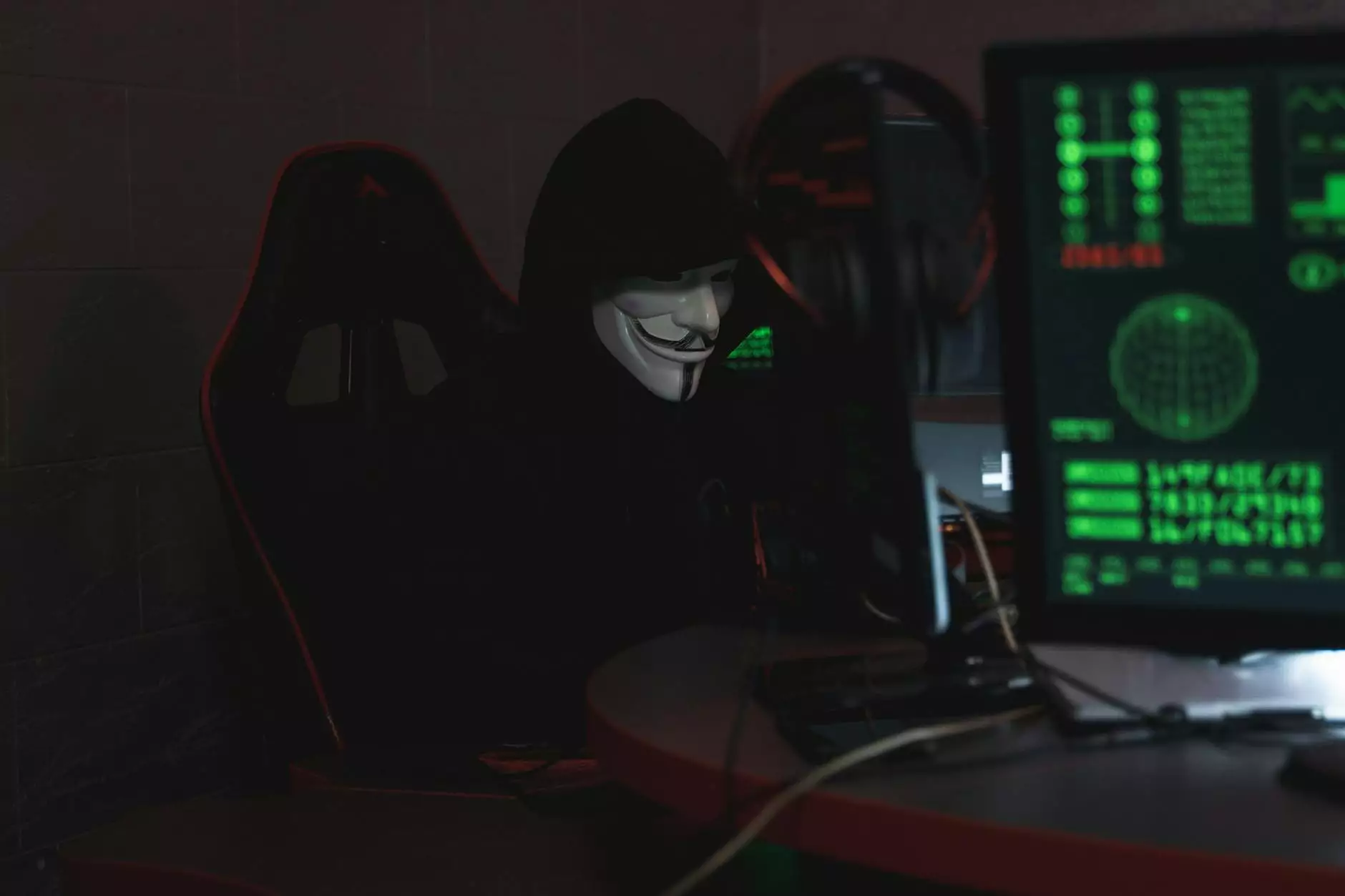
In today’s digital landscape, businesses face an unprecedented number of threats to their IT infrastructure. As cybercriminals evolve, traditional security measures often fail to keep pace. This is where malware machine learning comes into play, providing sophisticated solutions that not only detect but also predict malware attacks.
Understanding Malware and Its Evolution
Malware, or malicious software, refers to any software intentionally designed to cause damage to a computer, server, or computer network. Traditionally, malware was simplistic—often identifiable by identifiable signatures. However, as technology has advanced, so has the complexity of malware.
Today, we encounter variants, such as:
- Viruses: Attach themselves to clean files and spread throughout a computer system.
- Trojan Horses: Disguise themselves as legitimate software, tricking users into installing them.
- Ransomware: Encrypts files and demands a ransom for decryption.
- Spyware: Secretly obtains information by transmitting data from the hard drive to the fraudster.
The Need for Machine Learning in Cybersecurity
As the sophistication of malware increases, businesses require more advanced tools for detection and prevention. Machine learning, a subset of artificial intelligence, enables systems to learn from past experiences and improve their predictive capabilities over time. This technology is crucial in the face of:
- Increasing Malware Variants: New types of malware emerge daily, making it challenging for antivirus solutions to keep up.
- Zero-Day Exploits: Attacks that occur on the same day a vulnerability is discovered, often before it has been patched.
- Polymorphic Malware: Malware that changes its code to avoid detection.
How Malware Machine Learning Works
Malware machine learning utilizes algorithms to analyze vast quantities of data related to software behavior, user patterns, and system vulnerabilities. Here's how it operates:
Data Collection and Preprocessing
First, data from various sources is collected, including signature files, network logs, and user activity. This data is then preprocessed to remove inconsistencies and irrelevant information.
Feature Extraction
The next step is to extract relevant features that can help distinguish between benign and malicious software. Features may include:
- File Size
- Executable Code Patterns
- System Calls
- Network Activity
Model Training
Using labeled datasets (malicious vs. benign), machine learning algorithms are trained to recognize patterns in the data. Common algorithms used include:
- Support Vector Machines (SVM)
- Decision Trees
- Neural Networks
Real-Time Detection and Response
Once trained, these models can be applied in real-time to analyze incoming data streams, helping to detect and mitigate threats instantly.
Benefits of Implementing Malware Machine Learning in Your Business
Businesses like Spambrella are leading the charge in utilizing malware machine learning for robust cybersecurity. The advantages of implementing this technology include:
1. Enhanced Detection Rates
Machine learning algorithms can analyze and detect malware with higher accuracy than traditional methods, significantly reducing false positives and missed detections.
2. Predictive Capabilities
By continually learning from new data, machine learning systems can predict potential threats, allowing businesses to take preventative measures before attacks occur.
3. Reduced Response Time
Automated responses enabled by machine learning can drastically reduce the time to contain and mitigate threats, minimizing damage.
4. Cost Efficiency
Although there is an initial investment in deploying machine learning solutions, the long-term savings from reduced downtimes and breach costs are substantial.
Case Study: Spambrella’s Success with Malware Machine Learning
Spambrella has successfully implemented a malware machine learning framework that has revolutionized its IT services and security systems. Here’s a brief overview of the outcomes:
Implementation Process
Spambrella initiated the process by:
- Conducting a comprehensive audit of existing security measures.
- Collecting historical attack data.
- Training machine learning models using this data.
Results Achieved
- Improved Malware Detection: Detection rates increased by 95%, significantly reducing false positives.
- Decreased Response Time: Automated threat responses cut incident resolution times in half.
- Enhanced Customer Trust: Increased customer confidence in service security boosted retention rates.
Challenges and Considerations
While the advantages of malware machine learning are compelling, businesses must also prepare for some challenges:
Data Privacy Concerns
Ensuring that data is handled appropriately is paramount. Companies must adhere to data protection regulations, such as GDPR.
Algorithm Bias
If training data is biased, the machine learning model may produce skewed results, affecting detection accuracy. Continuous monitoring and updating of training datasets are necessary.
Integration with Existing Systems
Implementing machine learning tools can require integration efforts with existing IT and security systems, which may pose a short-term operational challenge.
Future Trends in Malware Machine Learning
The field of malware machine learning is rapidly evolving, and several trends are emerging that could shape the future of cybersecurity:
1. Improved Algorithms
As computational power increases and more sophisticated algorithms are developed, our ability to detect malware will continue to improve.
2. Collaborative Defense Mechanisms
Firms may begin collaborating to share data on threats and attack patterns, enhancing the overall learning environment for machine learning models.
3. Proactive Security Postures
Organizations will increasingly shift from reactive to proactive security strategies, utilizing machine learning to anticipate and neutralize threats before they manifest.
4. Integration of AI with IoT Security
As IoT devices proliferate, machine learning solutions will become essential in protecting these environments from malware attacks.
Conclusion
The integration of malware machine learning into cybersecurity strategies represents a significant advancement in the protection of IT services. Embracing these technologies allows companies like Spambrella to stay ahead of emerging threats and safeguard their digital infrastructure.
By harnessing the power of machine learning, businesses not only enhance their security measures but also optimize operational efficiencies, ensuring that they remain resilient in the face of constant cyber threats. As the cyber landscape continues to evolve, machine learning will play an increasingly pivotal role in the way we protect our digital assets.