Mastering Labeling Tools for Machine Learning
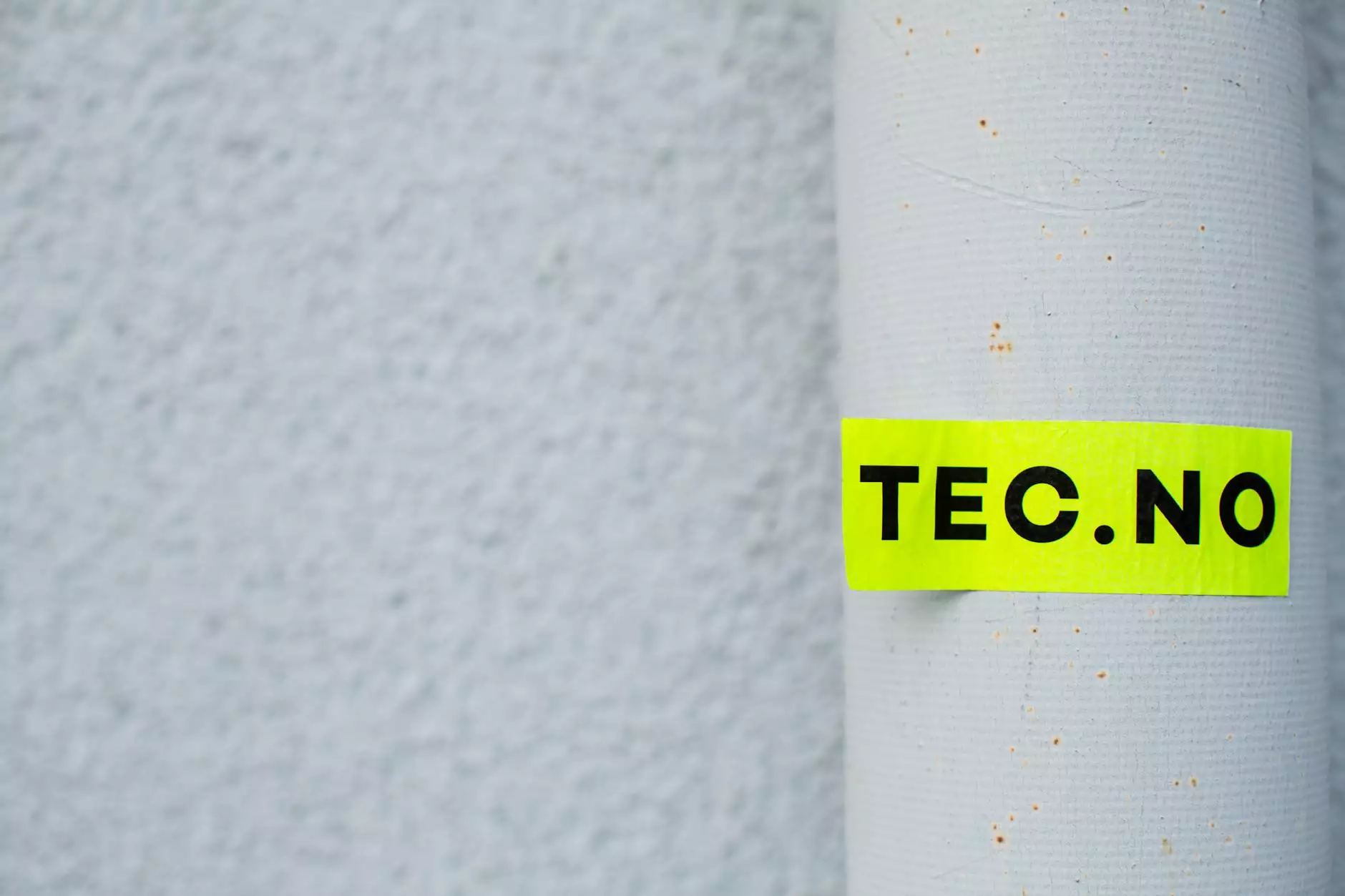
Labeling tools for machine learning are an essential part of the artificial intelligence ecosystem, acting as the bedrock upon which machine learning models are built. As businesses increasingly integrate AI into their operations, understanding and utilizing effective labeling tools has become critical. In this article, we will delve into the significance of data annotation, various types of labeling tools, and how Keylabs.ai can help propel your machine learning projects to success.
Understanding Data Annotation and its Importance
Data annotation refers to the process of labeling data, which is a crucial step in training machine learning models. This involves tagging data with relevant information, allowing the machine to learn from examples. A well-annotated dataset is the foundation for building accurate and reliable machine learning models.
The importance of data annotation cannot be overstated. It directly influences the performance of machine learning algorithms, enabling them to recognize patterns and make predictions. Effective labeling tools for machine learning make this process efficient and scalable, ensuring that vast amounts of data can be processed without compromising quality.
The Role of Labeling Tools in Machine Learning
Labeling tools serve several purposes in the realm of machine learning:
- Efficiency: Automating parts of the data labeling process significantly reduces the time required to prepare datasets.
- Accuracy: High-quality labeling tools minimize human errors, ensuring the labels are correct and consistent.
- Collaboration: Many tools allow teams to work together seamlessly, making it easier to share insights and improve annotation quality.
- Scalability: As businesses grow, the volume of data increases. Robust labeling tools can handle large datasets efficiently.
Key Features of Effective Labeling Tools
When selecting labeling tools for machine learning, key features should be considered:
- User-Friendly Interface: A simple, intuitive design that allows users to navigate easily.
- Multiple Annotation Types: Support for various data formats such as images, text, and audio.
- Integration Capabilities: Ability to integrate with other tools and platforms seamlessly.
- Quality Control Mechanisms: Built-in features that help ensure high annotation quality through review processes.
- Customizability: Flexibility to adapt the tool to specific project needs and workflows.
Top Labeling Tools for Machine Learning
1. Keylabs.ai: A Leading Data Annotation Platform
Keylabs.ai stands out as a premier data annotation platform designed to cater to diverse machine learning needs. Its features include:
- Advanced Collaboration: Keylabs.ai supports teams working simultaneously, enhancing productivity and ensuring uniformity in data labeling.
- AI-Assisted Annotation: Leveraging AI, the platform speeds up the annotation process while maintaining accuracy.
- Comprehensive Quality Assurance: Regular quality checks ensure that the data meets industry standards before being fed into machine learning models.
- Wide Range of Annotation Types: Supports bounding boxes, polygons, semantic segmentation, and more, adapting to your project’s requirements.
2. Labelbox
Labelbox is a robust tool that offers extensive functionalities, including:
- Custom Workflows: Users can create workflows tailored to specific project needs, improving the annotation process.
- Integration with Popular Frameworks: Works with frameworks like TensorFlow and PyTorch, creating a streamlined pipeline from annotation to model training.
3. Supervisely
Supervisely is a versatile platform known for:
- 3D and 2D annotation: Offers capabilities for annotating both 3D objects and traditional 2D images.
- Built-In Visualization Tools: Users can visualize their annotations directly in the platform, ensuring accuracy as they work.
Adopting Labeling Tools in Your Business
With the surge in machine learning applications across industries, incorporating labeling tools for machine learning into your business strategy can yield significant benefits. Here’s how:
1. Identify Your Needs
Assess your current data processes and identify areas where labeling tools can enhance productivity and accuracy.
2. Choose the Right Tool
Evaluate different tools based on the features discussed earlier, and consider conducting trial runs to determine which tool best fits your team's workflow.
3. Train Your Team
Ensure that your team goes through comprehensive training on how to use the chosen labeling tool effectively. Offer continuous learning opportunities to keep them updated with new features.
4. Monitor and Adjust
After implementation, monitor the process continuously. Gather feedback from your team and make necessary adjustments to workflows and processes for improved efficiency.
Case Studies: Success with Labeling Tools
1. Retail and E-commerce
A leading retail company implemented Keylabs.ai to annotate product images for an AI-powered recommendation system. By utilizing the platform's collaborative features, the team reduced their data preparation time by 40%, leading to faster model deployment and improved sales.
2. Healthcare Diagnostics
A healthcare startup focused on developing a diagnostic tool for medical images utilized advanced labeling features to train their models accurately. The annotation process enabled them to reach 95% accuracy in diagnoses, significantly enhancing patient care outcomes.
Future Trends in Data Annotation
As machine learning continues to evolve, so do the tools we use for data annotation. Some trends to watch for include:
- Increased Automation: AI will play a larger role in automating data labeling, making processes faster and more efficient.
- Enhanced Collaboration Features: Future tools will prioritize enhanced collaboration functionalities for teams spread across different regions.
- Quality Assurance Innovations: New mechanisms to ensure high-quality annotations before data reaches the model training phase will become increasingly important.
Conclusion
In conclusion, the landscape of labeling tools for machine learning is rich and diverse, offering businesses vital resources to enhance their AI projects. With platforms like Keylabs.ai, organizations can efficiently annotate data, ensuring high-quality inputs for machine learning models.
Embracing these tools not only boosts your machine learning capabilities but also positions your business as a forward-thinking entity ready to leverage the power of AI. It’s time to take your data annotation efforts to the next level!